Vulnerability is managed using data science models. Aside from limited modifications related to model structure, such as designing and hyper-boundary tuning, there are a variety of other components that can aid in successful model execution.
In this post, we’ll learn about the five most important elements to communicate with stakeholders in order to build assumptions and prepare them to deal effectively with the consequences that an information science group produces.
Causation vs. relationship
It’s common for commercial clients to want to know what the primary goal of data science models is. In any case, data science models incorporating machine learning (ML) rely on predictive analysis, which does not determine causality. Causation vs. relationship.
It’s common for corporate clients to want to know the basic explanation for a data science model’s outcome. In any event, predictive analytics is used in data science models that involve machine learning (ML), which does not determine causality.
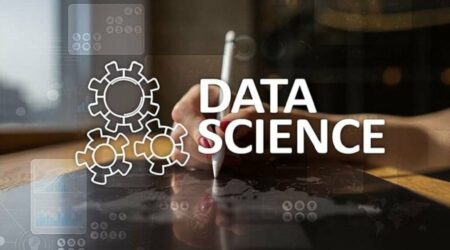
Periodic vs. continuous training
For business applications where there is a lot of approaching information and a requirement for models to gradually learn quick changes in the examples — for example, financial exchange expectation, which includes persistently moving business sector information — continuously preparing ML models is beneficial.
When information conditions are relatively static and slow, preparing ML models is sometimes sufficient. There may be a heritage design that is learned when a model is initially designed to use a large amount of data.
False positives vs. false negatives: what’s the difference?
Tuning the model toward fewer false up-sides for further developing model accuracy is best when a customer’s time is valuable and they simply need to be advised of the best return estimates. In some circumstances, the business end customer can’t afford to miss out on an opportunity, in which case adjusting the model toward fewer false negatives for future model development is beneficial.
A balance between accuracy and review is desirable in most cases, as an excessive number of false positives can indicate client weakness, and an excessive number of spurious negatives can undermine model credibility.
Business errors vs. modelling
Errors in modelling are to be expected in any measured learning process. However, there is another set of errors that are characterised as business blunders. Although these are not technically incorrect, the business client may perceive them as mistakes.
Balanced versus unbalanced data
When working with grouping models, it’s critical to understand how classes are distributed across the total population. The actual information may be used while obtaining new prepared information to prepare a clever information science model, as an educated authority (SMEs) can combine a summary of the classes together. In any case, it’s critical to understand the amount of verifiable data that each class contains.
Follow and connect with us on Facebook, LinkedIn & Twitter