Artificial intelligence and machine learning techniques have already proven their effectiveness in pharmaceutical processes. Drug detection is one of the important processes to identify new drug candidates in the fields of medicine, biotechnology and pharmacology. According to the new FDA, there are five stages of the development of a new drug. These include detection and development, preliminary research, clinical research, FDA review and post-FDA marketing safety monitoring. Because drug discovery requires so much data and research, many pharmaceutical companies are adopting artificial intelligence and machine learning to speed up drug discovery.
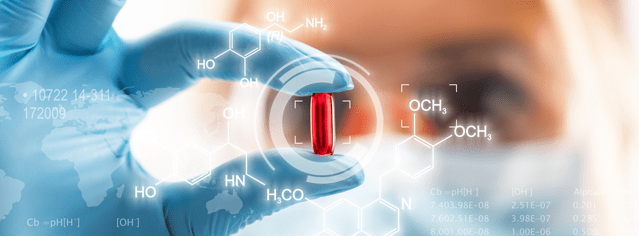
AI and ML techniques can also reduce drug development costs. Drug detection is a data-based procedure. It includes large-scale data such as high-resolution medical images, genomic profiles, metabolites, molecular structures, and biological information. Machine learning and artificial intelligence, which work through in-depth study, can interconnect, synchronize, and link existing data to help detect patterns in data groups.
Since drugs can only function based on their affinity for target proteins in the body, it is analyzed that drug adherence is a major obstacle in the detection and detection process. New research in chemistry and machine learning will reduce that barrier. Deepbar can calculate the relationship between drug candidates and their targets. Deepbar Mix traditional chemical calculations with recent advances in machine learning. It correctly computes the binding free energy, but previous methods require only a fraction of the calculations required.
“Bar” in Deepbar means “Bennett acceptance rate”. This is a decade old algorithm that used inaccurate link free energy calculations. According to the researchers, Deepbar can accelerate drug discovery and protein engineering.
According to the study, using the Bennett acceptance rate usually requires knowledge of two “endpoint” conditions. Knowledge of a drug molecule associated with a protein and a drug molecule is completely different from a protein, as well as several intermediate states, viz. Different levels of partial binding, all of which reduce computational speed.
New machine learning techniques reduce intermediate states by applying a Bennett access rate to the so-called machine learning framework called Deep Generation Models. According to Fischer-Lubac Professor Bin Zhang, a professional development professor of chemistry at MIT and co-author of a new paper describing the technology, these models form a reference condition for each endpoint, bounded state, and asynchronous state.
Using depth-generating modelling, researchers borrowed from the field of computer vision. Although the adoption of computer vision with chemistry was a major invention of Deepbar, the crossover also posed some challenges. “These models were originally developed for 2D imaging,” Xinjiang Ding says. “But here we have proteins and molecules, which is really a 3D structure. Therefore, in our case the adoption of these methods was the biggest technical challenge that we faced ”.
In experiments using small protein-like molecules, Deepbar estimated that free energy is 50 times faster than previous methods. Researchers have started thinking about using it for drug testing, especially in the context of COVID. In addition to drug discovery, they believe that DeepBar will help in protein design and engineering because this method can be used for communication between multiple proteins. They also plan to improve new machine learning techniques in the future, so as to be made possible by recent advances in computer science.
Follow and connect with us on Facebook, LinkedIn & Twitter