There is a slew of reasons why a new AI project might never get off the ground. Aside from the obvious examples of sceptics with low expectations, there are a number of concerns that are less evident but just as dangerous.
Many projects fail because the people in charge have no idea what they’re doing. Some projects fail because their developers are unsure how to proceed when there are more difficulties than solutions. The following is a list of five of the most common reasons that AI initiatives fail.
Poor data management
Data management issues can derail an AI project faster than anything else. Sanitized data must be labelled (and labelled appropriately) and documented.
What this means is that a standard should be established early on to ensure that data is efficiently collected, saved, and maintained. This contains variables such as the data’s ‘meaning’ so that it can be used to train models. These procedures should be set in stone at the start of a project and should not change over time.
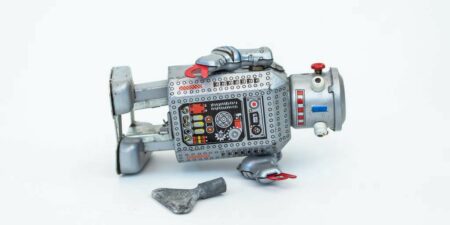
Lack of clear business objectives
Because they don’t have clear business objectives, AI projects frequently fail to take off. The traditional organisation will find it difficult to focus on a quantifiable business aim first, rather than designing a tool or system to tackle a problem.
What this means is that AI projects frequently begin as a novel shiny item with little link to what generates commercial value. Before beginning an AI project, the following questions should be asked:
- “Can you tell me about the business challenge you’re seeking to solve?”
- “How will artificial intelligence (AI) be applied to solve this business problem in a meaningful way?”
If you can’t answer these questions, your project probably doesn’t have a clear business goal. You should cease writing code if this is the case. Instead of wasting time on a project that is unlikely to assist the company gain income, you should concentrate on adequately defining a clear goal.
Lack of governance and standards
If there isn’t sufficient governance and standards in place, AI initiatives may be doomed from the start. These must be defined in the ‘early days’ of the project to avoid risks such as misconfiguration, security flaws, or incompatibility.
What this means is that AI projects must establish governance and standards early on to avoid being doomed from the start due to procedural issues. It’s worth repeating that one of AI’s flaws is its inability to be explained. Any algorithmic conclusion must be able to be ‘explained’ in a way that a business line owner understands and accepts. This will necessitate the establishment of suitable governance and standards from the start.
Lack of leadership commitment and ownership
What this means is that without qualified expertise available or dedicated to an AI project, considerable progress is improbable. An AI project can only flourish if it has capable leaders who are dedicated to its success.
 Follow and connect with us on Facebook, LinkedIn & Twitter